Ethical Challenges in AI-based Penetration Testing: Key Insights
Artificial Intelligence (AI) has revolutionized cybersecurity, offering powerful tools for protecting digital assets. However, as AI-based penetration testing emerges as a cutting-edge approach to identifying vulnerabilities, it brings many ethical challenges that cannot be ignored. The intersection of AI and security testing raises critical questions about privacy, consent, and potential unintended consequences.
As organizations rush to implement AI-driven security measures, they often overlook the ethical implications of these advanced systems. AI’s ability to autonomously probe networks and systems for weaknesses presents a double-edged sword: while it can significantly enhance security postures, it also introduces risks of overreach and unauthorized access. This dilemma leaves security professionals grappling with a crucial question: How can we harness the power of AI in penetration testing without compromising ethical standards?
This blog post will delve into the complex ethical challenges in AI-based penetration testing. We’ll explore this innovative approach’s fundamental concepts, examine its ethical concerns, and discuss the legal and regulatory hurdles that organizations must navigate. Moreover, we’ll investigate strategies for striking a delicate balance between robust security practices and ethical considerations, ultimately providing insights on addressing these challenges in an increasingly AI-driven cybersecurity world.
Understanding AI-based Penetration Testing
Definition and Key Components
AI-based penetration testing integrates artificial intelligence and machine learning algorithms into security assessment processes. This advanced approach automates vulnerability detection, exploitation, and reporting. Key components include intelligent scanning tools, adaptive learning systems, and autonomous decision-making modules.
Benefits over Traditional Methods
AI-driven penetration testing offers significant advantages compared to conventional methods. It provides faster and more comprehensive coverage of complex systems, reduces human error, and continuously adapts to emerging threats. This approach enables real-time threat detection and response, enhancing overall security posture.
Current Applications in Cybersecurity
In the cybersecurity landscape, AI-based penetration testing is being deployed across various domains. It is utilized in network security to identify and exploit vulnerabilities in real-time. Web application security benefits from AI’s ability to detect complex attack patterns. Additionally, AI-powered tools are increasingly used in cloud security assessments, identifying misconfigurations and potential data breaches more efficiently than traditional methods.
As we delve deeper into the ethical implications of AI-based penetration testing, it’s crucial to understand the potential risks and challenges associated with this powerful technology.
Ethical Concerns in AI-driven Security Testing
Potential for Unauthorized Access
While powerful, AI-based penetration testing tools raise concerns about potential misuse. These systems can autonomously discover and exploit vulnerabilities, potentially granting unauthorized access to sensitive systems. The risk of these tools falling into malicious hands or being manipulated for nefarious purposes is a significant ethical concern.
Data Privacy Implications
AI-driven security testing often involves processing vast amounts of sensitive data. This raises questions about data handling, storage, and protection. Organizations must ensure compliance with data protection regulations and maintain the confidentiality of information gathered during testing processes.
Bias in AI Algorithms
AI algorithms used in penetration testing may inadvertently inherit biases from their training data or design. These biases could lead to overlooking certain vulnerabilities or disproportionately focusing on specific types of systems, potentially leaving critical security gaps unaddressed.
Accountability and Responsibility Issues
Determining responsibility for actions taken by AI systems during penetration testing can be complex. If an AI-driven test causes unintended damage or disruption, questions arise about who bears the liability – the AI system’s developers, the organization conducting the test, or the AI itself. This ambiguity in accountability poses significant ethical and legal challenges.
As we consider these ethical concerns, it becomes clear that addressing them is crucial for the responsible development and deployment of AI in security testing.
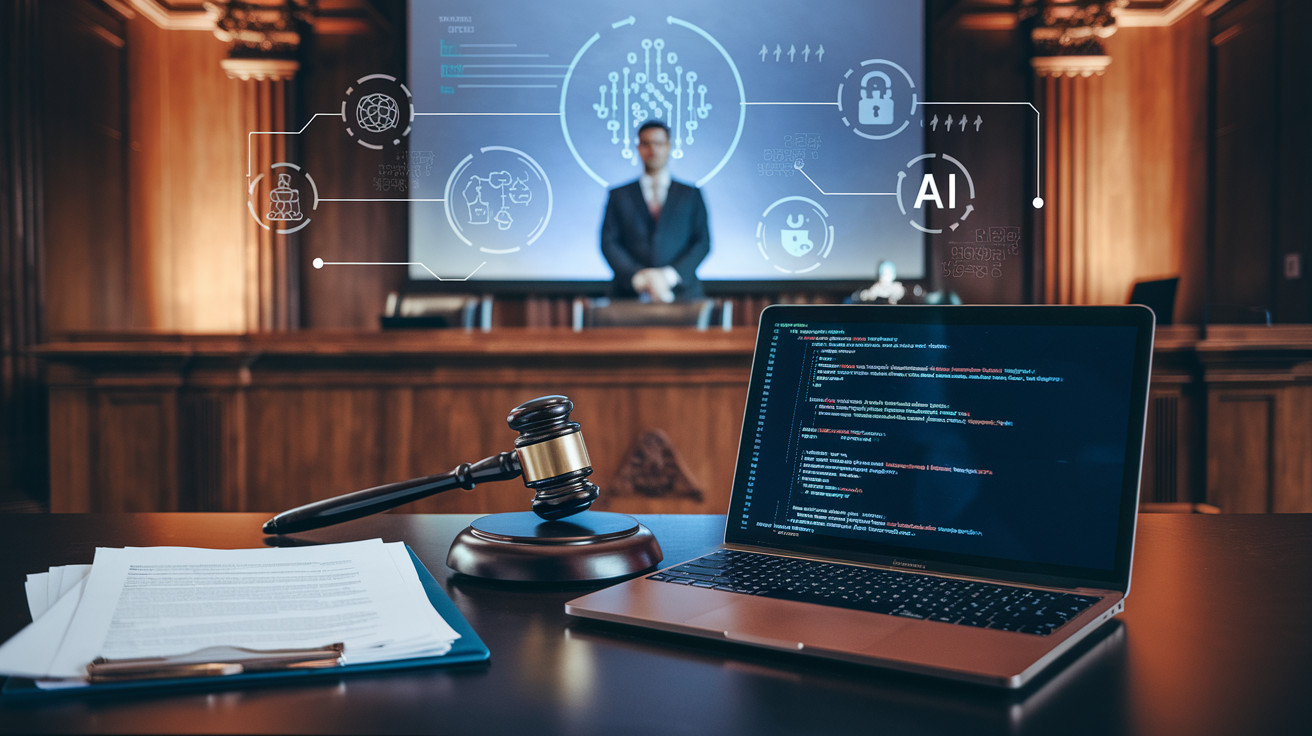
Legal and Regulatory Challenges
A. Compliance with existing cybersecurity laws
AI-based penetration testing must adhere to existing cybersecurity laws, which can vary significantly across jurisdictions. Organizations must ensure their AI systems operate within legal boundaries, respecting data protection regulations and privacy laws. This includes obtaining consent for testing activities and safeguarding sensitive information discovered during the process.
B. International legal considerations
As AI-driven security testing often transcends national borders, organizations face complex international legal challenges. Countries have varying regulations on AI usage, data protection, and cybersecurity practices. Navigating this intricate web of laws requires careful consideration and expert legal guidance to avoid potential violations and legal disputes.
C. Liability in case of unintended consequences
The autonomous nature of AI systems in penetration testing raises questions about liability when unintended consequences occur. Determining responsibility becomes complicated if an AI-driven test causes unintended damage or disruption to a target system. Organizations must establish clear protocols and liability frameworks to address potential issues arising from AI-based testing activities.
These legal and regulatory challenges underscore the need for a comprehensive approach to AI-based penetration testing. As we explore this field further, we’ll examine how to strike a balance between robust security measures and ethical considerations.
Balancing Security and Ethics
Establishing clear boundaries for AI testing
Setting well-defined boundaries is crucial in AI-based penetration testing. Organizations must clearly delineate the scope of AI systems’ operations, specifying which systems and data they can access. This involves creating detailed testing protocols that outline permissible actions and areas strictly off-limits. By establishing these boundaries, companies can ensure that AI tools do not inadvertently breach sensitive areas or cause unintended damage.
Implementing safeguards and fail-safes
Robust safeguards and fail-safe mechanisms are essential to maintaining ethical standards while leveraging AI for security testing. These measures include implementing kill switches that can immediately halt AI operations if unintended behavior is detected. Additionally, organizations should establish multi-layered approval processes for critical actions and incorporate human oversight at key decision points. Regular audits and real-time monitoring of AI activities further enhance safety and ethical compliance.
Ensuring transparency in AI decision-making processes
Transparency in AI-driven penetration testing is vital for maintaining trust and accountability. Organizations should strive to make AI decision-making processes as clear and interpretable as possible. This involves documenting the AI’s logic, providing detailed logs of its actions, and ensuring that security professionals can understand and explain the rationale behind AI-generated findings. By prioritizing transparency, companies can address concerns about AI bias and build confidence in the ethical use of these advanced tools.
Addressing Ethical Challenges
Developing ethical guidelines for AI penetration testing
To address the ethical challenges in AI-based penetration testing, organizations must establish comprehensive guidelines. These guidelines should outline the boundaries of AI system behavior, emphasizing the importance of respecting data privacy and system integrity. Key considerations include defining permissible actions, setting limits on data collection, and ensuring transparency in testing processes.
Training AI systems with ethical considerations
Incorporating ethical considerations into AI training is crucial. This involves designing algorithms prioritizing ethical decision-making and programming AI systems to recognize and avoid potentially harmful actions. By instilling ethical values during training, AI penetration testing tools can operate within acceptable boundaries while maintaining effectiveness.
Regular audits and assessments of AI testing methods
Implementing a system of regular audits and assessments helps maintain ethical standards in AI-based penetration testing. These evaluations should examine the AI’s performance, decision-making processes, and adherence to established ethical guidelines. Continuous monitoring allows for timely identification and correction of any ethical deviations.
Collaboration between ethicists and cybersecurity professionals
Fostering collaboration between ethicists and cybersecurity experts is essential for addressing ethical challenges. This interdisciplinary approach ensures that ethical considerations are seamlessly integrated into the technical aspects of AI-based penetration testing. Organizations can develop more robust and ethically sound testing methodologies by combining expertise.
Conclusion
AI-based penetration testing presents a powerful tool for identifying and addressing cybersecurity vulnerabilities. However, this technology brings forth significant ethical challenges that must be carefully considered. From potential misuse of AI systems to unintended consequences of autonomous testing, organizations must navigate a complex landscape of ethical, legal, and regulatory issues.
A balanced approach is crucial to addressing these challenges. Organizations should implement robust governance frameworks, adhere to ethical guidelines, and maintain human oversight in AI-driven security testing processes. By prioritizing transparency, accountability, and responsible use of AI technologies, the cybersecurity industry can harness the benefits of AI-based penetration testing while mitigating ethical risks and maintaining public trust.
Call to Action
We invite you to share your thoughts and experiences in the comments section. Your insights and feedback are valuable in fostering a collaborative discussion on enhancing security measures. By engaging, you agree to our Privacy Policy.
Subscribe to our monthly newsletter and follow us on our Facebook, X, and Pinterest channels for more insights and updates on cybersecurity trends and best practices. Our blog provides valuable information and resources to help you stay informed and prepared against evolving threats.
Engage with our community to share knowledge, ask questions, and stay connected with industry developments. Visit our About Us page to learn more about who we are and what we do. Furthermore, please reach out through our Contact Us page if you have any questions. You can also explore our Services to discover how we can help enhance your security posture.
Frequently Asked Questions
AI-based penetration testing involves using artificial intelligence and machine learning algorithms to identify system vulnerabilities. Unlike traditional methods, it automates scanning, exploitation, and reporting processes, enabling faster and more adaptive security assessments.
Key ethical challenges include:
1. Privacy Violations: Potential misuse of sensitive data during automated testing.
2. Unauthorized Access: AI tools might unintentionally exploit vulnerabilities beyond authorized scopes.
3. Algorithm Bias: AI systems may overlook or disproportionately focus on certain vulnerabilities.
4. Accountability: Determining who is responsible for unintended consequences caused by AI tools.
AI-based penetration testing offers several advantages over traditional methods:
1. Faster and more comprehensive scans of complex systems.
2. Continuous learning to adapt to new threats.
3. Reduced human error in detecting vulnerabilities.
4. Real-time threat detection and response capabilities.
Organizations can address these challenges by:
1. Defining Boundaries: Setting clear protocols for AI tool operations.
2. Implementing Safeguards: Using kill switches and requiring human oversight.
3. Ensuring Transparency: Documenting AI decision-making processes and maintaining clear logs.
4. Ethical Training: Developing AI systems with ethical considerations in mind.
Yes, AI-based penetration testing must comply with existing cybersecurity laws and data protection regulations. Challenges include navigating:
1. Jurisdictional Variations: Different countries have varying laws on AI and cybersecurity.
2. Liability: Determining accountability for unintended damages caused by AI tools. Organizations should consult legal experts to ensure compliance and mitigate risks.